Stochastic Processes on Graphs 2015/16
Lecturer: Dr. Anton Klimovsky Contact
Time & Place: Wednesdays, 14-16 Uhr @ Raum WSC-N-U-4.04
Prerequisites: Probability Theory II
Target Audience: Master Math.
Load: 2 hrs/week (+ a Seminar)
Start: 21. Oktober
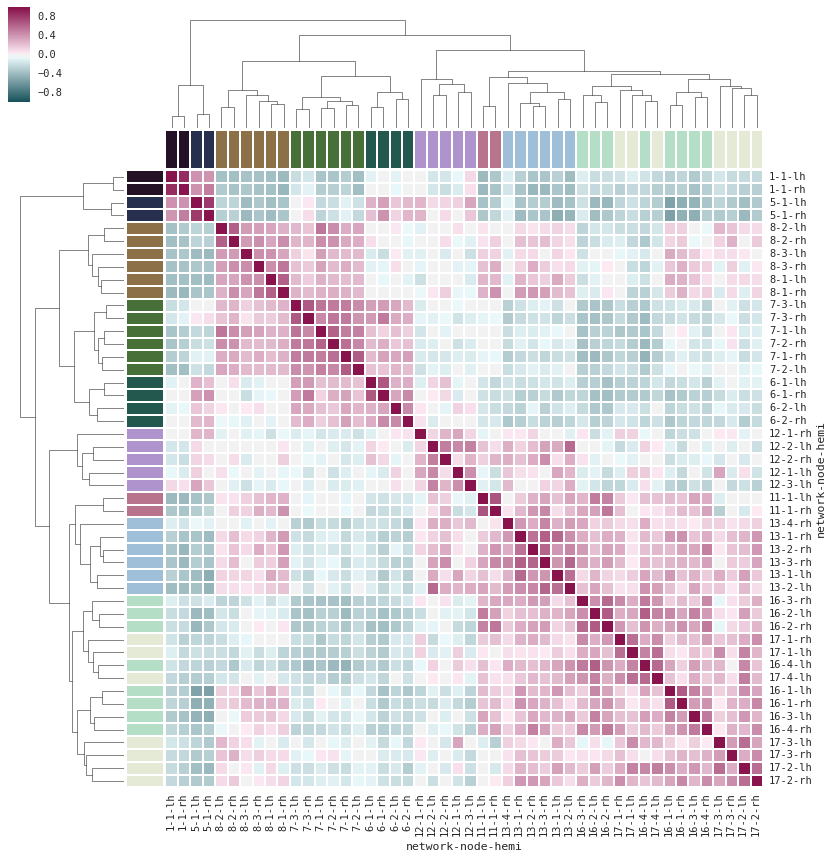
Stochastic Processes on Graphs
Over the past decade, networks became an extremely popular modeling paradigm. Here, behind each complex system, one often tries to find a network ($\approx$ graph) which encodes the interactions between the system's components.
In this course, we will get acquainted with the models of stochastic processes on graphs and methods to analyze them. We will deal with models, where the system's local variables live on the vertices of the graph. These local variables interact via the graph edges. While some of the ideas and terminology come from theoretical physics, such models are fundamental mathematical objects and are wide-spread in statistics, computer science, life sciences, etc.
We will learn about replica symmetry breaking, extremal processes, cavity method, local weak convergence, and (time permitting) message passing, Thouless-Anderson-Palmer (TAP) equations.
The course is followed by a concentrated seminar on the course topics (leading to 6 ECTS points).
Topics:
- A short glimpse into Statistical Physics: Curie-Weiss model, Ising-like models on graphs, phase transitions, order parameters, mean-field equations, ground states, Gibbs measures, pure states, variational principles.
- Models with inhomogeneous interactions: range-free spin glasses, REM, GREM, Sherrington-Kirkpatrick model, Parisi theory, ultrametricity, Gibbs measures, variational principles.
- (Time permitting) Inference on/of networks: message passing, TAP-like equations.
Class by class readings
- Lecture 1:
- Markov random fields. Gibbs distributions. Hammersley-Clifford theorem: Bremaud, Theorem 7.2.2, Winkler, Theorem 3.3.2.
- Lecture 2:
- Statistical Physics formalism: Klimovsky. Chapter 1, Mézard, Montanari. Chapter 2.
- Lecture 3:
- Curie-Weiss model: Bolthausen. Section 1.4, Bovier. Section 1.3, Dembo, Montanari. Section 1.1.
- Graphical models: Dembo, Montanari. Section 1.1.
- Lecture 4:
- Sherrington-Kirkpatrick model: Bolthausen. Section 3.2, Bovier. Section 2.
- Gaussian comparison inequality Klimovsky. Section 2.1, Bovier. Section 5.1.
- Lecture 5:
- Gaussian concentration inequality, self-averaging of the log-partition function, superadditivity of the log-partition function. Bolthausen. Section 3.1, Bovier. Section 5.2.
- Lecture 6:
- Aizenman-Sims-Starr extended variational principle. Aizenman, Sims, Starr. Mean-Field Spin Glass models from the Cavity-ROSt Perspective. 2007, Bovier. Section 5.3.
- Lecture 7:
- Aizenman-Sims-Starr's representation. The lower bound for the log-partition function. Aizenman, Sims, Starr. Mean-Field Spin Glass models from the Cavity-ROSt Perspective. 2007
- Limiting Gibbs measures, sampling convergence. [Panchenko]
- Lecture 8:
- Limiting Gibbs measures, sampling convergence. [Panchenko]
- A mathematical formulation of the Parisi Ansatz. [Panchenko]
- Lecture 9:
- Parisi's formula.
- Ruelle's probability cascades.
- Lecture 10:
- Ruelle's probability cascades as scaling limits of the GREM weights.
- Ghirlanda-Guerra identities.
- Lecture 11:
- Distribution of the limiting Gibbs measure via ultrametricity and Ghirlanda-Guerra identities.
- Stochastic stability: Aizenman-Contucci's, Bolthausen-Sznitman's, and Panchenko's.
Literature
-
Math:
- Statistical Mechanics of Disordered Systems: A Mathematical Perspective. Cambridge University Press. Bovier. 2006.
- The Sherrington-Kirkpatrick Model. Springer. Panchenko. 2013.
- Statistical Mechanics and Algorithms on Sparse and Random Graphs. Notes. Montanari.
- Mean Field Models for Spin Glasses. Vol. I & II. Springer. Talagrand. 2011.
-
Physics:
Research articles ($\approx$ seminar topics):
- Contact me.